-
Notifications
You must be signed in to change notification settings - Fork 268
New issue
Have a question about this project? Sign up for a free GitHub account to open an issue and contact its maintainers and the community.
By clicking “Sign up for GitHub”, you agree to our terms of service and privacy statement. We’ll occasionally send you account related emails.
Already on GitHub? Sign in to your account
Curious about Glow implementation: some weights look frozen? #20
Comments
This is interesting, but unfortunately I have no idea for this. Do you have some minimal example of code to run or test like small model with fake input data ? Are you sure you are plotting the right thing? |
Thank you very much for the reply! I just tried visualizing the weights in the provided notebook
If I understand your suggestion correctly, I will try to reduce the flow's complexity (number of steps etc.) and plot the weights again. Maybe on MNIST instead of CelebA... |
Yes good idea, maybe it would be better to start from much more smaller learning rate and simpler architecture. MNIST is good idea also. Can you check this ? |
Though I'm not too sure if it's going to be useful, let me share with you what I encountered while playing with the code for CelebA 64x64. Actually, at that time, I changed the learning dynamics carelessly from the original: I started to run the training with num_steps=1000 and lr_ph: 0.0005 from the beginning, and it turned out that this change caused NaN error after all. In short, the warm-up strategy, that is, In [33] and In [34] for example in Celeba64x64_22steps.ipynb, seems important. |
@geosada thanks for your comment 👍 |
Thanks a lot @geosada for pointing out the importance of the warm-up strategy! Although I have heard of starting with a small learning rate that goes up and down again, but in
|
I also noticed that the CelebA notebook uses a per-pixel loss
while for MNIST, it's the sum over all pixels Maybe that’s why the l2 loss goes up in the MNIST notebook, because the loss summed over all pixels overpowers the l2 loss: Furthermore, in the official GLOW implementation, But when I tried to divide the MNIST loss by This was with Also another unrelated thought--I wonder if act norm, if it acts like batch norm, will interact with l2 regularization to produce adaptive learning rate. |
Hi, I believe I was playing with LR rate manually, so there is no specific reason for this schedule. When experimenting in jupyter notebooks, I usually start from small LR e.g. 0.0001 and test model for few epochs to check whether it trains or not. If so, I increase LR and then systematically decrease e.g. [0.001, 0.0005, 0.0001, 0.00005]. This time I increased LR at the end, probably because I noted that model is not learning fast enough, so I wanted to help it, or maybe this is just a typo. Sorry for the inconvenience. |
Wow, you're doing a great detective work :)
I believe you should work with smaller LR. NFs are very complicated networks with many parts which are very fragile and they don't train fast (at least from my experience). If you have a bad luck you can always sample some hard minibatch which will generate large loss and which will break whole training. You can try to overcome this problem with some gradient clipping techniques. Or maybe one could write some custom optimizer which would reject these updates for which loss is far from the current running mean. |
Hi Krzysztof,
When visualizing the distribution of weights and gradients of each tensor over training, I noticed that some the weights don't seem to be updating. E.g.
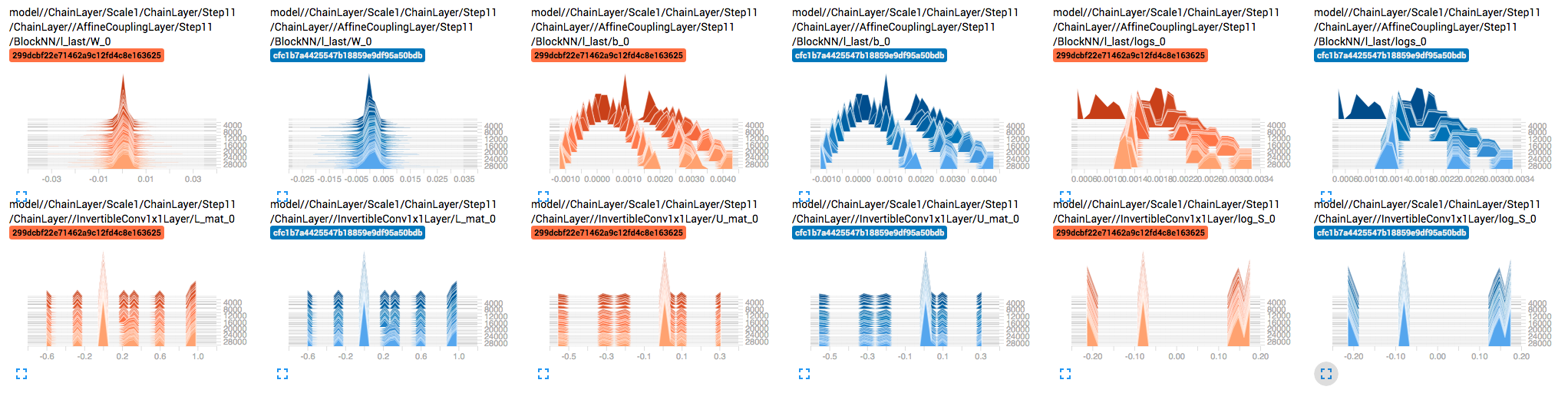
InvertibleConv1x1Layer
'sU_mat
,L_mat
, andlog_S
.My first thought was that maybe the gradients are too small, but it doesn't look like that's the case:
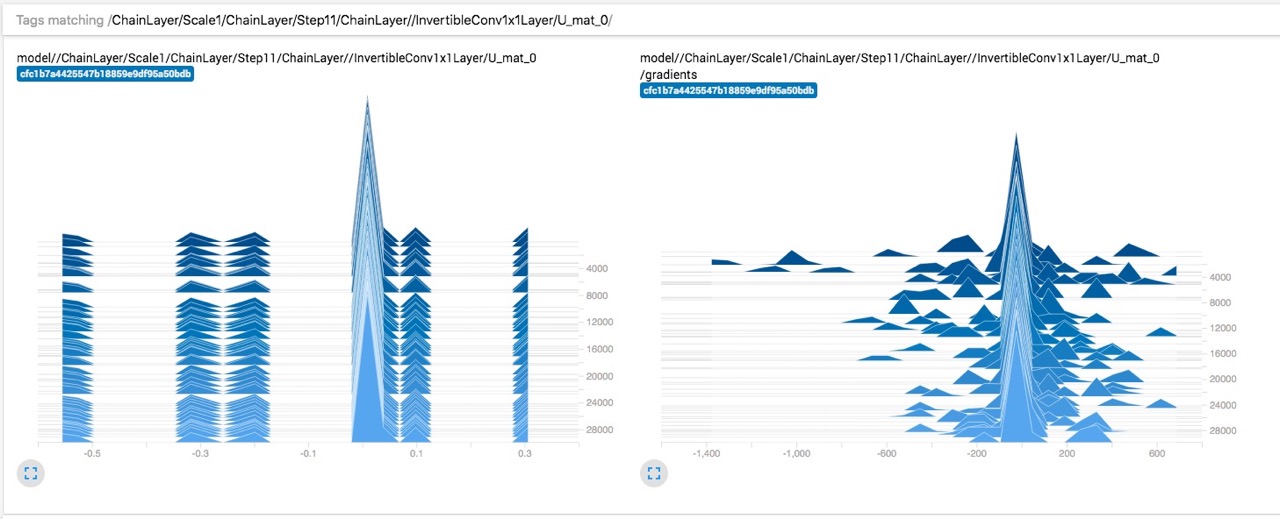
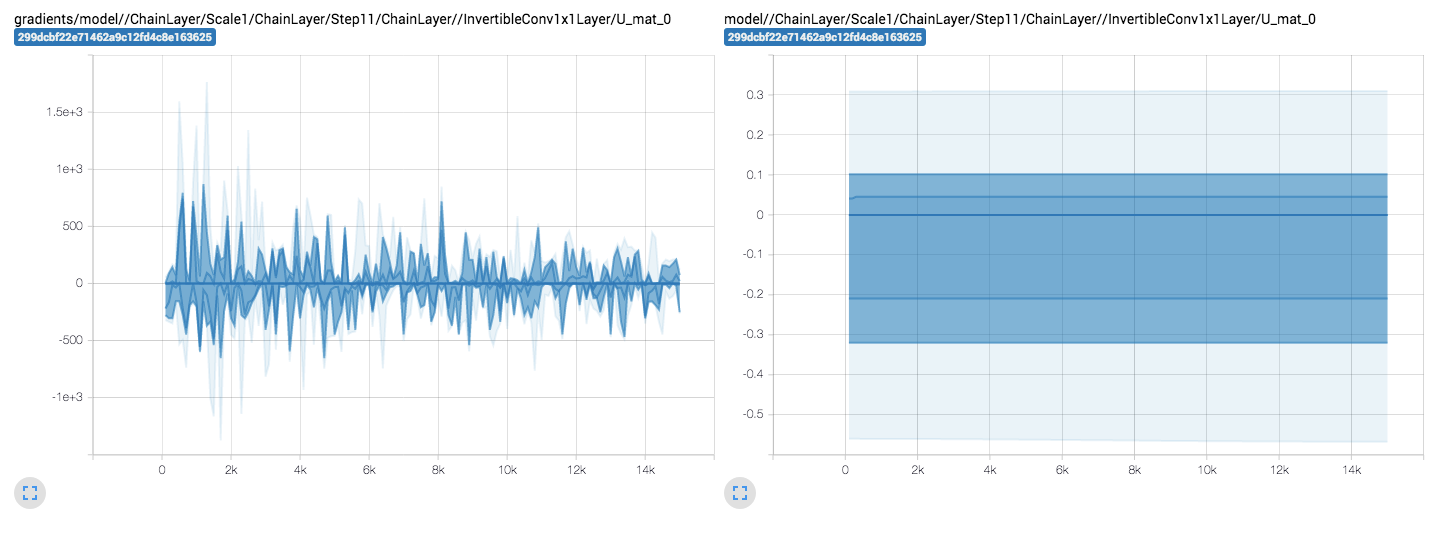
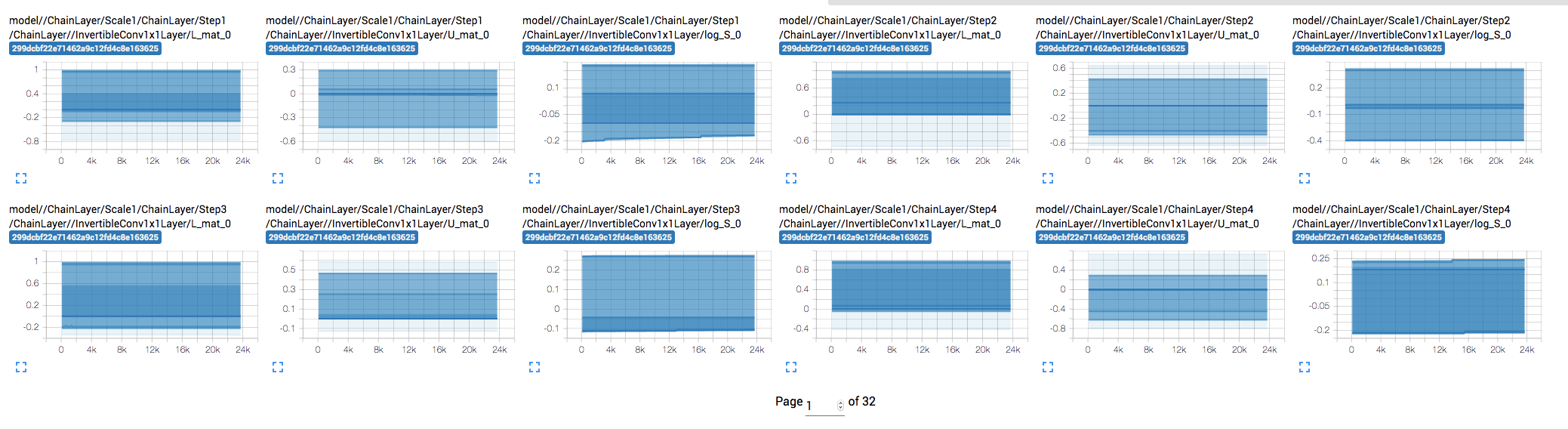
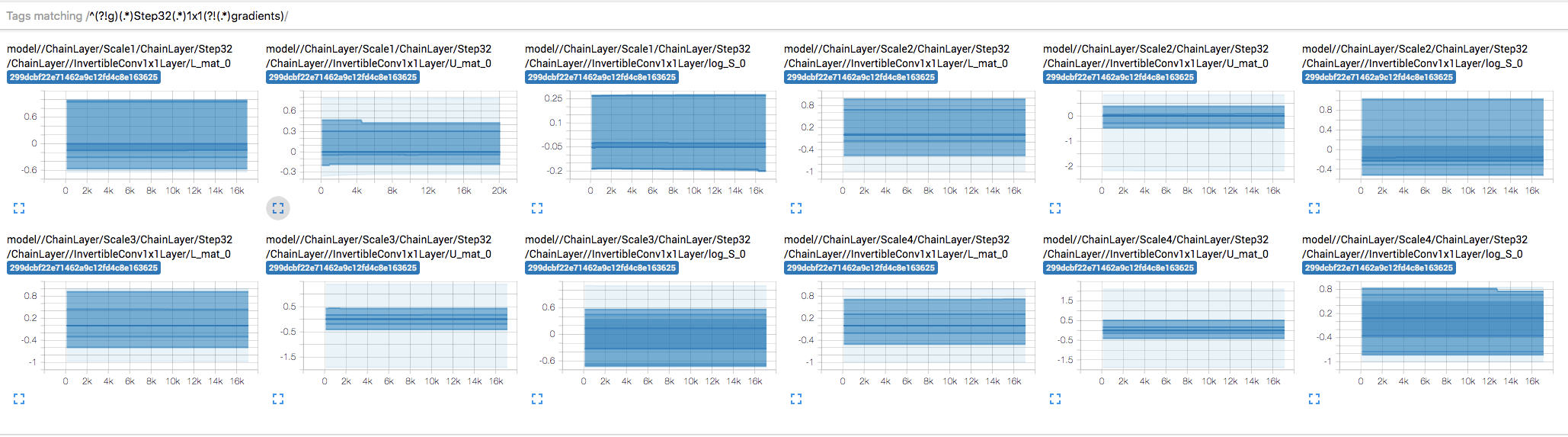
Weights remain mostly constant:
But gradients are... pretty explosive 😔
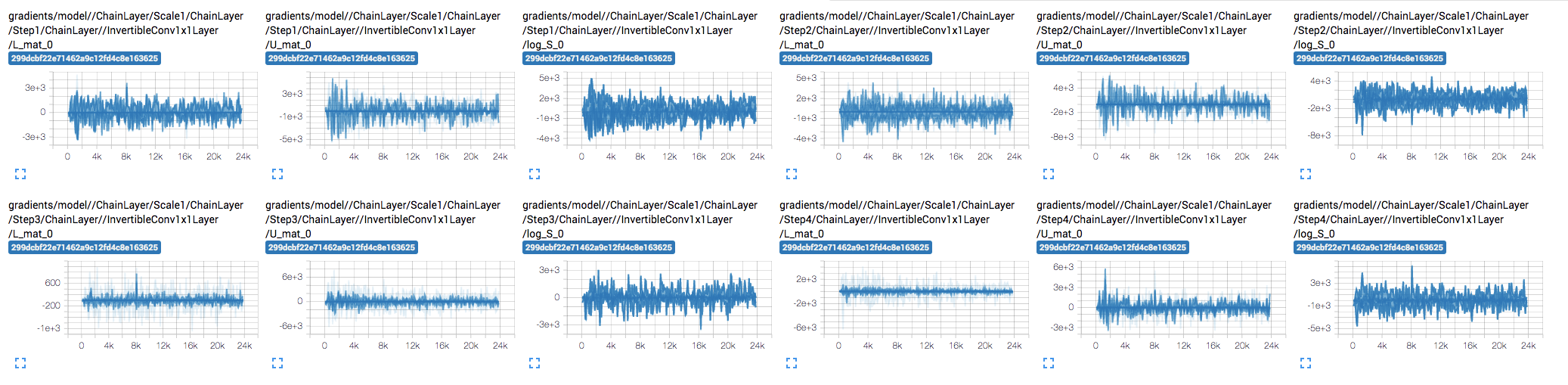
I didn't change the core code and used the high-level API, but trained it on a different task and it is plugged into a larger model.
I will try running the original example you provided and report back with that, but in the meantime I was wondering if you (or anyone else) had any early ideas about this. Thanks!
The text was updated successfully, but these errors were encountered: