forked from linogaliana/python-datascientist
-
Notifications
You must be signed in to change notification settings - Fork 0
/
Copy pathindex.qmd
366 lines (262 loc) · 12.7 KB
/
index.qmd
1
2
3
4
5
6
7
8
9
10
11
12
13
14
15
16
17
18
19
20
21
22
23
24
25
26
27
28
29
30
31
32
33
34
35
36
37
38
39
40
41
42
43
44
45
46
47
48
49
50
51
52
53
54
55
56
57
58
59
60
61
62
63
64
65
66
67
68
69
70
71
72
73
74
75
76
77
78
79
80
81
82
83
84
85
86
87
88
89
90
91
92
93
94
95
96
97
98
99
100
101
102
103
104
105
106
107
108
109
110
111
112
113
114
115
116
117
118
119
120
121
122
123
124
125
126
127
128
129
130
131
132
133
134
135
136
137
138
139
140
141
142
143
144
145
146
147
148
149
150
151
152
153
154
155
156
157
158
159
160
161
162
163
164
165
166
167
168
169
170
171
172
173
174
175
176
177
178
179
180
181
182
183
184
185
186
187
188
189
190
191
192
193
194
195
196
197
198
199
200
201
202
203
204
205
206
207
208
209
210
211
212
213
214
215
216
217
218
219
220
221
222
223
224
225
226
227
228
229
230
231
232
233
234
235
236
237
238
239
240
241
242
243
244
245
246
247
248
249
250
251
252
253
254
255
256
257
258
259
260
261
262
263
264
265
266
267
268
269
270
271
272
273
274
275
276
277
278
279
280
281
282
283
284
285
286
287
288
289
290
291
292
293
294
295
296
297
298
299
300
301
302
303
304
305
306
307
308
309
310
311
312
313
314
315
316
317
318
319
320
321
322
323
324
325
326
327
328
329
330
331
332
333
334
335
336
337
338
339
340
341
342
343
344
345
346
347
348
349
350
351
352
353
354
355
356
357
358
359
360
361
362
363
364
365
366
---
toc: false
sidebar: false
title-block-style: none
css: styles/homepage.css
listing:
id: sample-listings
contents:
- "content/getting-started/*.qmd"
- "content/manipulation/*.qmd"
- "!content/manipulation/03_geopandas_tutorial.qmd"
- "content/visualisation/*.qmd"
- "content/modelisation/*.qmd"
- "content/NLP/*.qmd"
- "content/git/*.qmd"
type: grid
sort: "number"
categories: true
#fields: [image, title, github, gitlab]
#template: cards.ejs
---
::: {#hero-banner .column-screen}
:::: {.grid .column-page}
::::: {.headline .g-col-lg-6 .g-col-12 .g-col-md-12}
:::::: {.content-visible when-profile="fr"}
::::::: h1
Python pour la _data science_ {{< fa brands python >}}
:::::::
::::::
:::::: {.content-visible when-profile="en"}
::::::: h1
Data science with Python {{< fa brands python >}}
:::::::
::::::
::::::: h2
[Lino Galiana](https://github.com/linogaliana/)
:::::::
```{=html}
<div style="text-align: right;">
<a class="github-button" href="https://github.com/linogaliana/python-datascientist" data-icon="octicon-star" data-size="large" data-show-count="true" aria-label="Star this website on Github">Star this website on Github</a><script async defer src="https://buttons.github.io/buttons.js"></script>
</div>
```
:::::: {.content-visible when-profile="fr"}
Site web du cours [*Python pour la data science*](https://www.ensae.fr/courses/1425-python-pour-le-data-scientist)
<a href="https://github.com/linogaliana/python-datascientist" class="github"><i class="fab fa-python"></i></a>, une introduction à `Python` pour
la deuxième année du cursus d'ingénieur de l'[`ENSAE`](https://www.ensae.fr/) (Master 1).
<br>
L'ensemble du contenu de ce groupe est librement disponible ici
ou sur [`Github`](https://github.com/linogaliana/python-datascientist)
<a href="https://github.com/linogaliana/python-datascientist" class="github"><i class="fab fa-github"></i></a> et peut être testé
sous forme de _notebooks_ `Jupyter`.
::::::
:::::: {.content-visible when-profile="en"}
Course website [*Python for Data Science*](https://www.ensae.fr/courses/1425-python-pour-le-data-scientist)
<a href="https://github.com/linogaliana/python-datascientist" class="github"><i class="fab fa-python"></i></a>, an introduction to `Python` for the second year of the engineering curriculum at [`ENSAE`](https://www.ensae.fr/) (Master 1).
<br>
All content of this group is freely available here or on [`Github`](https://github.com/linogaliana/python-datascientist)
<a href="https://github.com/linogaliana/python-datascientist" class="github"><i class="fab fa-github"></i></a> and can be tested in the form of `Jupyter` notebooks.
::::::
<br>
<details>
<summary>
:::::: {.content-visible when-profile="fr"}
Exemple avec l'introduction à `Pandas`
::::::
:::::: {.content-visible when-profile="en"}
Example with the introduction to `Pandas`
::::::
</summary>
```{ojs}
//| echo: false
html`${printBadges({fpath: "content/manipulation/02_pandas_intro.qmd"})}`
```
</details>
:::::: {.content-visible when-profile="fr"}
<details>
<summary>
Au programme:
</summary>
Globalement, ce cours propose un contenu très complet pouvant autant
satisfaire des débutants en
_data science_ que des personnes à la recherche de contenu plus avancé :
<br>
1. __Manipulation de données__ : manipulation de données standards (`Pandas`), données géographiques (`Geopandas`), récupération de données (webscraping, API)...
1. __Visualisation de données__ : visualisations classiques (`Matplotlib`, `Seaborn`), cartographie, visualisations réactives (`Plotly`, `Folium`)
1. __Modélisation__ : _machine learning_ (`Scikit`), économétrie
1. __Traitement de données textuelles__ (NLP): découverte de la tokenisation avec `NLTK` et `SpaCy`, modélisation...
1. **Introduction à la _data science_ moderne** : _cloud computing_, `ElasticSearch`, intégration continue...
L'ensemble du contenu de ce site s'appuie sur des __données
ouvertes__, qu'il s'agisse de données françaises (principalement
issues de la plateforme
centralisatrice [`data.gouv`](https://www.data.gouv.fr) ou du site
_web_ de l'[Insee](https://www.insee.fr)) ou de données
américaines. Le programme est présenté de manière linéaire dans la partie supérieure de cette page (👆️) ou de manière désordonnée ci-dessous (👇️).
Un bon complément du contenu du site web est le cours que nous donnons avec Romain Avouac en dernière année de l'ENSAE plus tourné autour de la mise en production de projets _data science_ : [https://ensae-reproductibilite.github.io/](https://ensae-reproductibilite.github.io/website/)
</details>
::::::
:::::: {.content-visible when-profile="en"}
<details>
<summary>
On the agenda:
</summary>
Overall, this course offers a comprehensive content that can satisfy both beginners in data science and those looking for more advanced material:
<br>
1. **Data Manipulation**: standard data manipulation (`Pandas`), geographic data (`Geopandas`), data retrieval (web scraping, APIs)...
1. **Data Visualization**: classic visualizations (`Matplotlib`, `Seaborn`), cartography, reactive visualizations (`Plotly`, `Folium`)
1. **Modeling**: machine learning (`Scikit`), econometrics
1. **Text Data Processing** (NLP): introduction to tokenization with `NLTK` and `SpaCy`, modeling...
1. **Introduction to Modern Data Science**: cloud computing, `ElasticSearch`, continuous integration...
All content on this site relies on open data, whether it is French data (mainly from the central platform [`data.gouv`](https://www.data.gouv.fr) or the Insee website) or American data. The program is presented linearly at the top of this page (👆️) or in a disordered manner below (👇️).
A good complement to the website content is the course given with Romain Avouac in the final year at ENSAE, which focuses more on the production of data science projects: [https://ensae-reproductibilite.github.io/](https://ensae-reproductibilite.github.io/website/)
</details>
::::::
:::::
::::: {.g-col-lg-6 .g-col-12 .g-col-md-12}
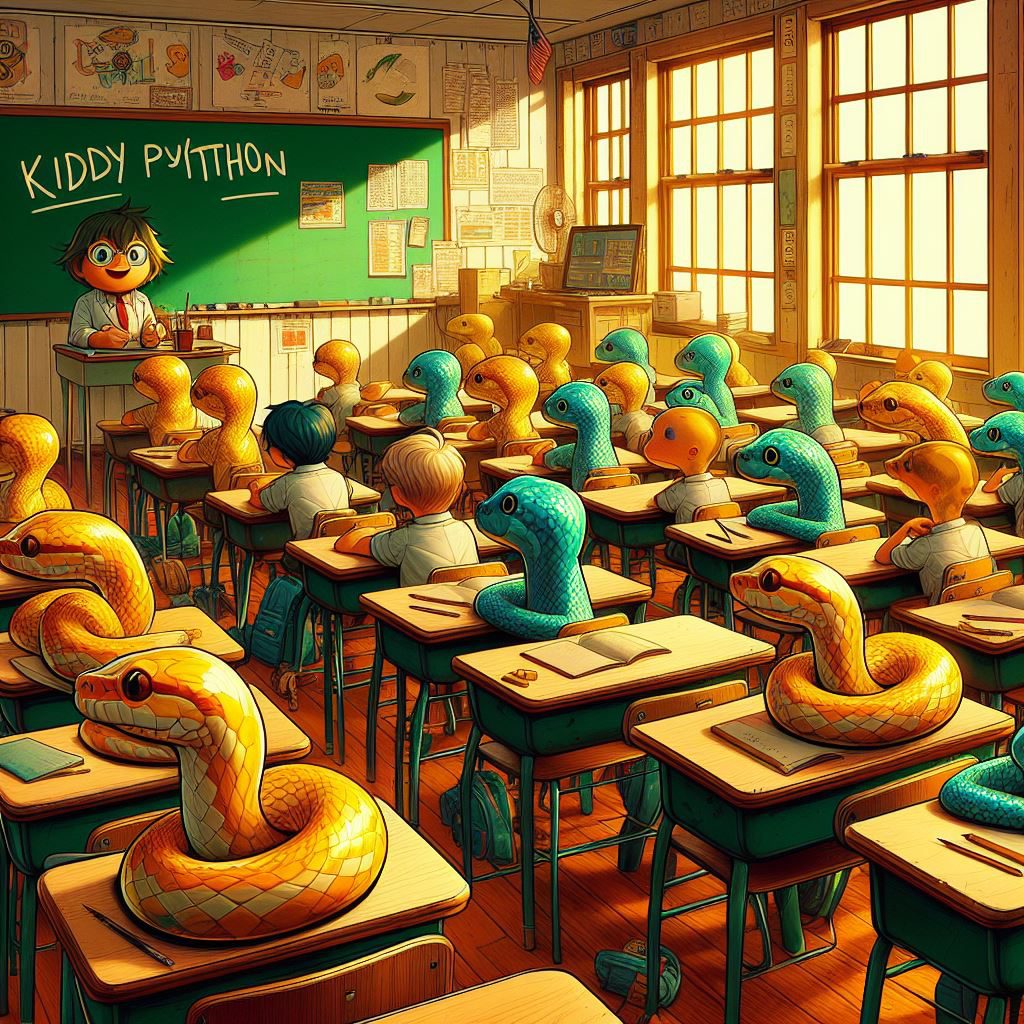
:::::
::::
:::
<br>
## Thèmes en vrac {.unnumbered}
Pour découvrir `Python` {{< fa brands python >}} de manière désordonnée. La version ordonnée est dans la partie supérieure de cette page (👆️).
::: {#sample-listings}
:::
```{python}
#| include: false
#| eval: false
import os
import glob
import yaml
list_files = glob.glob("./content/**/*.qmd", recursive=True)
posts = [l for l in list_files if l.endswith('.qmd') ]
def extract_slug_from_markdown(file_path):
with open(file_path, 'r') as file:
content = file.read()
front_matter = yaml.safe_load(content.split('---', 2)[1])
if isinstance(front_matter, dict) and 'slug' in front_matter:
slug = front_matter['slug']
return slug
return None
# Extract the slugs
slugs = {post: extract_slug_from_markdown(post) for post in posts if extract_slug_from_markdown(post) is not None}
# Lines to insert in a Netlify _redirect file
redirects = [f"/{slug} /posts/{post}" for slug, post in zip(slugs, posts)]
# Write the _redirect file
with open("_site/_redirects", "w") as f:
f.write("\n".join(redirects))
```
```{ojs}
//| echo: false
function reminderBadges({
sourceFile = "content/01_toto.Rmd",
type = ['md', 'html'],
split = null,
onyxiaOnly = false,
sspCloudService = "python",
GPU = false,
correction = false
} = {}) {
if (Array.isArray(type)) {
type = type[0];
}
let notebook = sourceFile.replace(/(.Rmd|.qmd)/, ".ipynb");
if (correction) {
notebook = notebook.replace(/content/, "corrections");
} else {
notebook = notebook.replace(/content/, "notebooks");
}
const githubRepoNotebooksSimplified = "github/linogaliana/python-datascientist-notebooks";
const githubAlias = githubRepoNotebooksSimplified.replace("github", "github.com");
const githubRepoNotebooks = `https://${githubAlias}`;
let githubLink ;
if (notebook === "") {
githubLink = githubRepoNotebooks;
} else {
githubLink = `${githubRepoNotebooks}/blob/main`;
}
const notebookRelPath = `/${notebook}`;
const [section, chapter] = notebook.split("/").slice(-2);
githubLink = `<a href="${githubLink}${notebookRelPath}" class="github"><i class="fab fa-github"></i></a>`;
const sectionLatest = section.split("/").slice(-1)[0];
const chapterNoExtension = chapter.replace(".ipynb", "");
const onyxiaInitArgs = [sectionLatest, chapterNoExtension];
if (correction) {
onyxiaInitArgs.push("correction");
}
const gpuSuffix = GPU ? "-gpu" : "";
const sspcloudJupyterLinkLauncher = `https://datalab.sspcloud.fr/launcher/ide/jupyter-${sspCloudService}${gpuSuffix}?autoLaunch=true&onyxia.friendlyName=%C2%AB${chapterNoExtension}%C2%BB&init.personalInit=%C2%ABhttps%3A%2F%2Fraw.githubusercontent.com%2Flinogaliana%2Fpython-datascientist%2Fmaster%2Fsspcloud%2Finit-jupyter.sh%C2%BB&init.personalInitArgs=%C2%AB${onyxiaInitArgs.join('%20')}%C2%BB`;
let sspcloudJupyterLink;
if (type === "md") {
sspcloudJupyterLink = `[](${sspcloudJupyterLinkLauncher})`;
} else {
sspcloudJupyterLink = `<a href="${sspcloudJupyterLinkLauncher}" target="_blank" rel="noopener"><img src="https://img.shields.io/badge/SSP%20Cloud-Lancer_avec_Jupyter-orange?logo=Jupyter&logoColor=orange" alt="Onyxia"></a>`;
}
if (split === 4) {
sspcloudJupyterLink += '<br>';
}
const sspcloudVscodeLinkLauncher = `https://datalab.sspcloud.fr/launcher/ide/vscode-${sspCloudService}${gpuSuffix}?autoLaunch=true&onyxia.friendlyName=%C2%AB${chapterNoExtension}%C2%BB&init.personalInit=%C2%ABhttps%3A%2F%2Fraw.githubusercontent.com%2Flinogaliana%2Fpython-datascientist%2Fmaster%2Fsspcloud%2Finit-vscode.sh%C2%BB&init.personalInitArgs=%C2%AB${onyxiaInitArgs.join('%20')}%C2%BB`;
let sspcloudVscodeLink;
if (type === "md") {
sspcloudVscodeLink = `[](${sspcloudVscodeLinkLauncher})`;
} else {
sspcloudVscodeLink = `<a href="${sspcloudVscodeLinkLauncher}" target="_blank" rel="noopener"><img src="https://img.shields.io/badge/SSP%20Cloud-Lancer_avec_VSCode-blue?logo=visualstudiocode&logoColor=blue" alt="Onyxia"></a>`;
}
if (split === 5) {
sspcloudVscodeLink += '<br>';
}
let colabLink;
if (type === "md") {
colabLink = `[](http://colab.research.google.com/${githubRepoNotebooksSimplified}/blob/main${notebookRelPath})`;
} else {
colabLink = `<a href="https://colab.research.google.com/${githubRepoNotebooksSimplified}/blob/main${notebookRelPath}" target="_blank" rel="noopener"><img src="https://colab.research.google.com/assets/colab-badge.svg" alt="Open In Colab"></a>`;
}
if (split === 7) {
colabLink += '<br>';
}
let vscodeLink;
if (type === "md") {
vscodeLink = `[](https://github.dev/linogaliana/python-datascientist-notebooks${notebookRelPath})`;
} else {
vscodeLink = `<a href="https://github.dev/linogaliana/python-datascientist-notebooks${notebookRelPath}" target="_blank" rel="noopener"><img src="https://img.shields.io/static/v1?logo=visualstudiocode&label=&message=Open%20in%20Visual%20Studio%20Code&labelColor=2c2c32&color=007acc&logoColor=007acc" alt="githubdev"></a></p>`;
}
const badges = [
githubLink,
sspcloudVscodeLink,
sspcloudJupyterLink
];
if (!onyxiaOnly) {
badges.push(colabLink);
}
let result = badges.join("\n");
if (type === "html") {
result = `<p class="badges">${result}</p>`;
}
if (onyxiaOnly) {
result = `${sspcloudJupyterLink}${sspcloudVscodeLink}`;
}
return result;
}
function printBadges({
fpath,
onyxiaOnly = false,
split = 5,
type = "html",
sspCloudService = "python",
GPU = false,
correction = false
} = {}) {
const badges = reminderBadges({
sourceFile: fpath,
type: type,
split: split,
onyxiaOnly: onyxiaOnly,
sspCloudService: sspCloudService,
GPU: GPU,
correction: correction
});
return badges
}
// Example usage:
// printBadges({ fpath: "content/getting-started/05_rappels_types.qmd", onyxiaOnly: false, split: 5, type: "html", sspCloudService: "python", GPU: false, correction: false });
```