diff --git a/README.md b/README.md
index 1a8fe56bbe..532d9bf546 100644
--- a/README.md
+++ b/README.md
@@ -39,7 +39,7 @@ $ pip install nni
To update NNI to the latest version, add `--upgrade` flag to the above commands.
-## NNI capabilities in a glance
+## NNI capabilities at a glance
@@ -220,20 +220,20 @@ To update NNI to the latest version, add `--upgrade` flag to the above commands.
## Contribution guidelines
-If you want to contribute to NNI, be sure to review the [contribution guidelines](https://nni.readthedocs.io/en/stable/notes/contributing.html), which includes instructions of submitting feedbacks, best coding practices, and code of conduct.
+If you want to contribute to NNI, be sure to review the [contribution guidelines](https://nni.readthedocs.io/en/stable/notes/contributing.html), which includes instructions for submitting feedback, best coding practices, and code of conduct.
We use [GitHub issues](https://github.com/microsoft/nni/issues) to track tracking requests and bugs.
Please use [NNI Discussion](https://github.com/microsoft/nni/discussions) for general questions and new ideas.
-For questions of specific use cases, please go to [Stack Overflow](https://stackoverflow.com/questions/tagged/nni).
+For questions about specific use cases, please go to [Stack Overflow](https://stackoverflow.com/questions/tagged/nni).
-Participating discussions via the following IM groups is also welcomed.
+Participating in discussions via the following IM groups is also welcomed.
|Gitter||WeChat|
|----|----|----|
|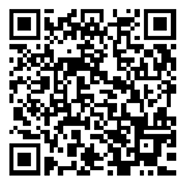| OR ||
Over the past few years, NNI has received thousands of feedbacks on GitHub issues, and pull requests from hundreds of contributors.
-We appreciate all contributions from community to make NNI thrive.
+We appreciate all contributions from the community to make NNI thrive.
@@ -266,15 +266,15 @@ We appreciate all contributions from community to make NNI thrive.
## Related Projects
-Targeting at openness and advancing state-of-art technology, [Microsoft Research (MSR)](https://www.microsoft.com/en-us/research/group/systems-and-networking-research-group-asia/) had also released few other open source projects.
+Targeting openness and advancing state-of-art technology, [Microsoft Research (MSR)](https://www.microsoft.com/en-us/research/group/systems-and-networking-research-group-asia/) has also released a few other open-source projects.
-* [OpenPAI](https://github.com/Microsoft/pai) : an open source platform that provides complete AI model training and resource management capabilities, it is easy to extend and supports on-premise, cloud and hybrid environments in various scale.
-* [FrameworkController](https://github.com/Microsoft/frameworkcontroller) : an open source general-purpose Kubernetes Pod Controller that orchestrate all kinds of applications on Kubernetes by a single controller.
-* [MMdnn](https://github.com/Microsoft/MMdnn) : A comprehensive, cross-framework solution to convert, visualize and diagnose deep neural network models. The "MM" in MMdnn stands for model management and "dnn" is an acronym for deep neural network.
-* [SPTAG](https://github.com/Microsoft/SPTAG) : Space Partition Tree And Graph (SPTAG) is an open source library for large scale vector approximate nearest neighbor search scenario.
-* [nn-Meter](https://github.com/microsoft/nn-Meter) : An accurate inference latency predictor for DNN models on diverse edge devices.
+* [OpenPAI](https://github.com/Microsoft/pai): an open-source platform that provides complete AI model training and resource management capabilities, it is easy to extend and supports on-premise, cloud, and hybrid environments in various scales.
+* [FrameworkController](https://github.com/Microsoft/frameworkcontroller): an open-source general-purpose Kubernetes Pod Controller that orchestrates all kinds of applications on Kubernetes by a single controller.
+* [MMdnn](https://github.com/Microsoft/MMdnn): A comprehensive, cross-framework solution to convert, visualize and diagnose deep neural network models. The "MM" in MMdnn stands for model management and "dnn" is an acronym for deep neural network.
+* [SPTAG](https://github.com/Microsoft/SPTAG): Space Partition Tree And Graph (SPTAG) is an open-source library for large-scale vector approximate nearest neighbor search scenarios.
+* [nn-Meter](https://github.com/microsoft/nn-Meter): An accurate inference latency predictor for DNN models on diverse edge devices.
-We encourage researchers and students leverage these projects to accelerate the AI development and research.
+We encourage researchers and students to leverage these projects to accelerate AI development and research.
## License
diff --git a/docs/source/feature_engineering/gbdt_selector.rst b/docs/source/feature_engineering/gbdt_selector.rst
index daded470b0..4217b49398 100644
--- a/docs/source/feature_engineering/gbdt_selector.rst
+++ b/docs/source/feature_engineering/gbdt_selector.rst
@@ -12,7 +12,7 @@ For now, we support the ``importance_type`` is ``split`` and ``gain``. But we wi
Usage
^^^^^
-First you need to install dependency:
+First you need to install the dependency:
.. code-block:: bash
@@ -32,8 +32,8 @@ Then
fgs = GBDTSelector()
# fit data
fgs.fit(X_train, y_train, ...)
- # get improtant features
- # will return the index with important feature here.
+ # get important features
+ # will return the index with an important feature here.
print(fgs.get_selected_features(10))
...
@@ -53,7 +53,7 @@ And you could reference the examples in ``/examples/feature_engineering/gbdt_sel
**lgb_params** (dict, require) - The parameters for lightgbm model. The detail you could reference `here `__
*
- **eval_ratio** (float, require) - The ratio of data size. It's used for split the eval data and train data from self.X.
+ **eval_ratio** (float, require) - The ratio of data size. It's used to split the eval data and train data from self.X.
*
**early_stopping_rounds** (int, require) - The early stopping setting in lightgbm. The detail you could reference `here `__.
@@ -62,9 +62,9 @@ And you could reference the examples in ``/examples/feature_engineering/gbdt_sel
**importance_type** (str, require) - could be 'split' or 'gain'. The 'split' means ' result contains numbers of times the feature is used in a model' and the 'gain' means 'result contains total gains of splits which use the feature'. The detail you could reference in `here `__.
*
- **num_boost_round** (int, require) - number of boost round. The detail you could reference `here `__.
+ **num_boost_round** (int, require) - number of boost rounds. The detail you could reference `here `__.
**Requirement of get_selected_features FuncArgs**
-* **topk** (int, require) - the topK impotance features you want to selected.
+* **topk** (int, require) - the topK important features you want to select.
diff --git a/docs/source/feature_engineering/gradient_feature_selector.rst b/docs/source/feature_engineering/gradient_feature_selector.rst
index 46bcf0ac6c..590d9746fc 100644
--- a/docs/source/feature_engineering/gradient_feature_selector.rst
+++ b/docs/source/feature_engineering/gradient_feature_selector.rst
@@ -24,29 +24,29 @@ Usage
...
X_train, X_test, y_train, y_test = train_test_split(X, y, test_size=0.33, random_state=42)
- # initlize a selector
+ # initialize a selector
fgs = FeatureGradientSelector(n_features=10)
# fit data
fgs.fit(X_train, y_train)
- # get improtant features
- # will return the index with important feature here.
+ # get important features
+ # will return the index with an important feature here.
print(fgs.get_selected_features())
...
-And you could reference the examples in ``/examples/feature_engineering/gradient_feature_selector/``\ , too.
+And you could reference the examples in ``/examples/feature_engineering/gradient_feature_selector/``\, too.
**Parameters of class FeatureGradientSelector constructor**
*
- **order** (int, optional, default = 4) - What order of interactions to include. Higher orders may be more accurate but increase the run time. 12 is the maximum allowed order.
+ **order** (int, optional, default = 4) - What order of interactions to include? Higher orders may be more accurate but increase the run time. 12 is the maximum allowed order.
*
**penalty** (int, optional, default = 1) - Constant that multiplies the regularization term.
*
- **n_features** (int, optional, default = None) - If None, will automatically choose number of features based on search. Otherwise, the number of top features to select.
+ **n_features** (int, optional, default = None) - If None, will automatically choose a number of features based on search. Otherwise, the number of top features to select.
*
**max_features** (int, optional, default = None) - If not None, will use the 'elbow method' to determine the number of features with max_features as the upper limit.
@@ -64,7 +64,7 @@ And you could reference the examples in ``/examples/feature_engineering/gradient
**shuffle** (bool, optional, default = True) - Shuffle "rows" prior to an epoch.
*
- **batch_size** (int, optional, default = 1000) - Nnumber of "rows" to process at a time.
+ **batch_size** (int, optional, default = 1000) - N number of "rows" to process at a time.
*
**target_batch_size** (int, optional, default = 1000) - Number of "rows" to accumulate gradients over. Useful when many rows will not fit into memory but are needed for accurate estimation.
@@ -94,10 +94,10 @@ And you could reference the examples in ``/examples/feature_engineering/gradient
*
- **X** (array-like, require) - The training input samples which shape = [n_samples, n_features]. `np.ndarry` recommended.
+ **X** (array-like, require) - The training input samples which shape = [n_samples, n_features]. `np.ndarray` recommended.
*
- **y** (array-like, require) - The target values (class labels in classification, real numbers in regression) which shape = [n_samples]. `np.ndarry` recommended.
+ **y** (array-like, require) - The target values (class labels in classification, real numbers in regression) which shape = [n_samples]. `np.ndarray` recommended.
*
**groups** (array-like, optional, default = None) - Groups of columns that must be selected as a unit. e.g. [0, 0, 1, 2] specifies the first two columns are part of a group. Which shape is [n_features].