You signed in with another tab or window. Reload to refresh your session.You signed out in another tab or window. Reload to refresh your session.You switched accounts on another tab or window. Reload to refresh your session.Dismiss alert
I'm currently doing a project work on INNs during my master study at university of applied science in Karlsruhe.
As an orientation I was using the toy implementation of the Gaußian Mixture Model.
I got strange results during my first approach as the reverse loss was rising extremely every few epochs - both on train and test data.
Further into the code, I've seen that the reverse_orig_loss is implemented with the MSE loss:
l_rev += lambd_predict * loss_fit(output_rev, x)
You write in the paper that the reverse loss has to be implemented with MMD. When I've changed the reverse loss to MMD, I got way better results and the loss is shrinking really well.
Is there a reason why you've used MSE at this point?
Thanks in advance!
The text was updated successfully, but these errors were encountered:
Hello,
I'm currently doing a project work on INNs during my master study at university of applied science in Karlsruhe.
As an orientation I was using the toy implementation of the Gaußian Mixture Model.
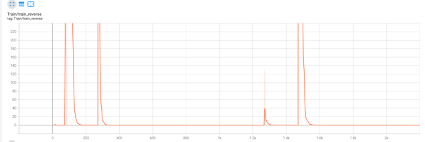
I got strange results during my first approach as the reverse loss was rising extremely every few epochs - both on train and test data.
Further into the code, I've seen that the reverse_orig_loss is implemented with the MSE loss:
You write in the paper that the reverse loss has to be implemented with MMD. When I've changed the reverse loss to MMD, I got way better results and the loss is shrinking really well.
Is there a reason why you've used MSE at this point?
Thanks in advance!
The text was updated successfully, but these errors were encountered: